The Rise of Agentic AI: How AI Agents, MCP, and A2A Are Shaping Enterprise Innovation
Agentic AI, MCPs, A2A: The New Enterprise Stack
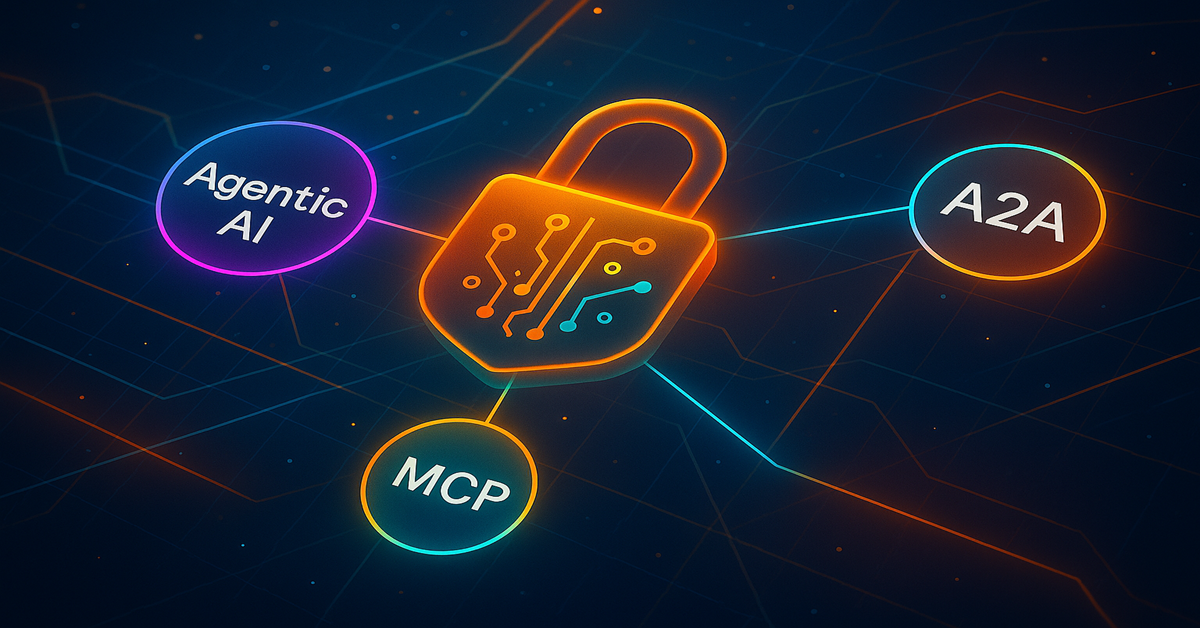
Introduction: Enterprise Innovation Beyond Traditional AI
In a landscape where speed, adaptability, and intelligence define winners, enterprises are moving beyond traditional AI. Static machine learning models and siloed automation no longer meet the demands of dynamic, multi-domain environments.
The future is unfolding through Agentic AI ecosystems — autonomous, collaborative AI agents operating with the support of Model Context Protocols (MCPs) and Agent-to-Agent (A2A) communication. This convergence is revolutionizing how enterprises innovate, operate, and compete globally.
If you are unfamiliar with foundational concepts like Agentic AI and MCPs, refer to our earlier posts:
Agentic Ecosystems: The New Blueprint for Enterprise AI
In contrast to legacy AI solutions that are created for standalone tasks, Agentic AI ecosystems are autonomous, modular, and contextually intelligent. They are composed of specialized AI agents—each with independent decision-making capabilities—operating in concert toward enterprise-wide objectives.
In practical terms, this means:
- Supply chain agents adjusting logistics dynamically.
- Financial agents autonomously optimizing investments.
- Product development agents coordinating faster go-to-market strategies.
This evolution empowers enterprises to create self-optimizing, resilient, and innovation-driven ecosystems.
MCP and A2A: The Core Enablers of Enterprise Intelligence
Deploying multiple AI agents introduces complexity. Collaboration among these agents demands not just communication, but a shared operational understanding. This is where Model Context Protocols (MCPs) and A2A Communication step in — not as optional tools, but as architectural necessities.
- MCP (Model Context Protocol): Establishes a standardized "mental model" among AI agents—aligning goals, rules, roles, and situational understanding.
- A2A (Agent-to-Agent Communication): Enables real-time negotiation, collaboration, and adjustment without human intervention, often leveraging the shared MCP foundation.
Rather than operating in silos, AI agents build collective intelligence, unlocking capabilities like swarm optimization, decentralized decision-making, and autonomous governance.
MCP vs A2A: Complementary, Not Competitive
Understanding the distinct roles of MCP and A2A is vital for enterprise architects.
Factor | Model Context Protocol (MCP) | Agent-to-Agent (A2A) Communication |
---|---|---|
Role | Defines shared understanding (rules, semantics) | Enables dynamic conversations/actions |
Focus | Agreement and context modeling | Execution and negotiation |
Nature | Static foundation, dynamic updates | Real-time, operational |
Example | Common definition of “urgent shipment” | Agents negotiating who will fulfill an order |
In essence:
- MCP defines the "language" and "terms of engagement."
- A2A is the active "dialogue" and "negotiation" based on those terms.
An enterprise without MCP risks fragmented agent behavior. An enterprise without A2A limits agents to siloed, manual interactions. Together, they create fluid, context-rich autonomous operations.
Driving Enterprise Innovation Through Agentic AI
Forward-looking enterprises are deploying agentic frameworks to unlock:
1. Autonomous Decision Networks
AI agents autonomously coordinate, negotiate, and act without central bottlenecks — crucial for supply chains, financial trading, and customer engagement operations.
2. Resilient Enterprise Architectures
Self-healing and self-optimizing systems where failures or disruptions are dynamically managed by interconnected agents without downtime.
3. Hyper-Personalization at Scale
AI agents tailor services for individual customers dynamically, using shared context and inter-agent coordination to provide real-time experiences.
For instance, Infoservices’ Generative AI Solutions support designing such decentralized, autonomous ecosystems built on robust data foundations and cloud-native infrastructures.
Strategic Shift: Why Enterprises Are Accelerating Adoption Now
Several macro-forces are pushing enterprises toward agentic architectures:
- Complexity Growth: Traditional rule-based automation is brittle and hard to scale across increasingly complex operations.
- Market Volatility: Enterprises need autonomous systems that adapt instantly to change.
- AI Democratization: Tools like Google Agent Development Kit simplify the creation of intelligent agents.
- Infrastructure Readiness: Cloud-native platforms (AWS, Azure, Databricks) make scaling agentic frameworks easier and more cost-effective.
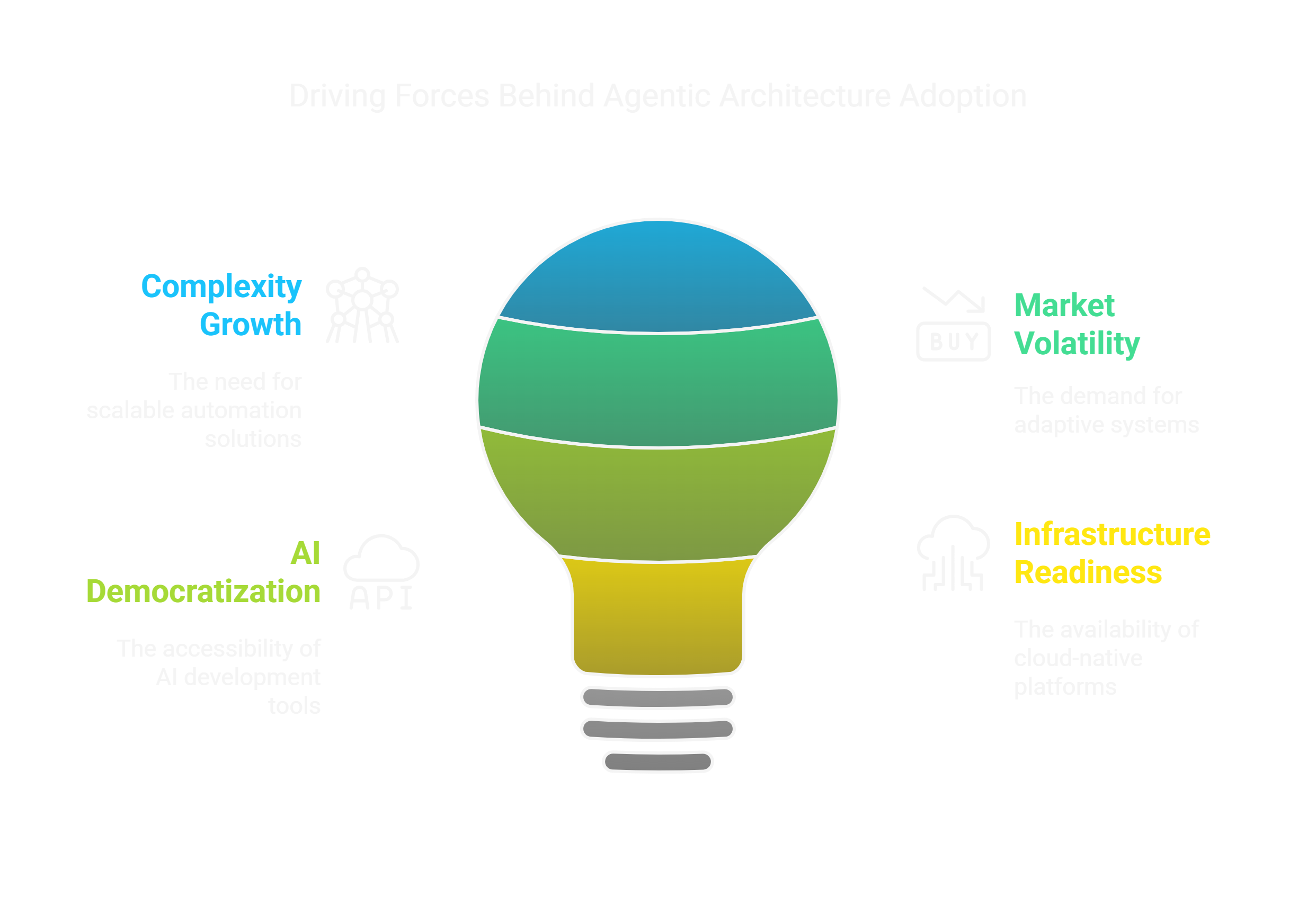
The Road Ahead: Toward Autonomous Enterprises
The convergence of Agentic AI, MCP, and A2A points toward a future where enterprises will:
- Self-regulate complex operations (logistics, finance, customer service) with minimal human intervention.
- Continuously innovate through autonomous, decentralized idea generation.
- Enhance resiliency and security via distributed agent intelligence.
At Infoservices, we help enterprises design, build, and scale intelligent agentic ecosystems across industries—integrating Generative AI, Cognitive AI, and Cloud Native solutions to deliver transformation at scale.
Conclusion: Architecting the Enterprise of the Future
Enterprises must move beyond isolated AI pilots and rigid automation to embrace true agentic intelligence—where autonomous agents dynamically collaborate across the value chain.
MCP and A2A aren’t just enablers; they are the foundation for creating resilient, adaptive, and innovative enterprises ready for an AI-first world.
Are you ready to evolve? Talk to Infoservices’ AI Experts about designing your agentic enterprise architecture today.
FAQs
Q1. How does agentic AI differ from traditional AI in enterprise use cases?
Agentic AI introduces autonomous decision-making and inter-agent collaboration, making enterprise operations more resilient and efficient compared to rule-based centralized AI systems.
Q2. What industries are adopting agentic AI fastest?
Financial services, manufacturing, logistics, and healthcare are leading the adoption due to the need for real-time decision-making and resilience.
Q3. What are some real-world examples of agent-to-agent communication?
Dynamic resource allocation in manufacturing plants, autonomous supply chain negotiations, and customer service ticket hand-offs between specialized agents.
Q4. How critical is security in agentic AI systems?
Extremely critical — without strong security measures, enterprises risk data breaches, compliance failures, and operational sabotage.
Q5. Where can I learn more about the basics of agentic AI?
Start with our Beginner’s Guide to Agentic AI.