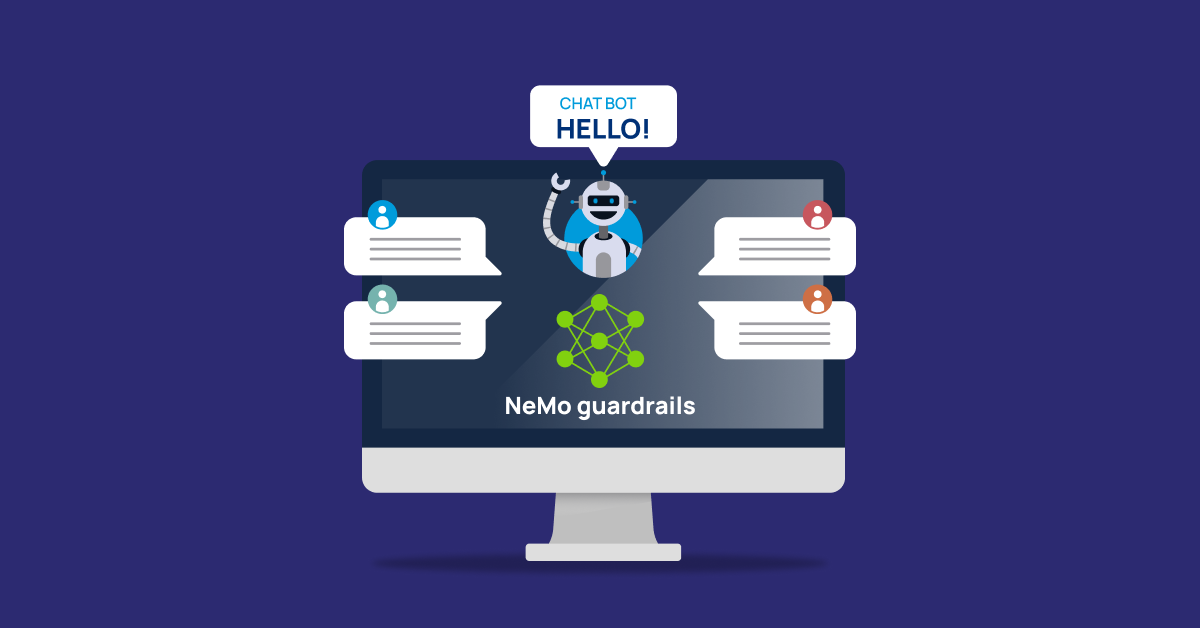
In this blog post, I will cover how we built a chatbot (using mock dataset) without prompt but using guardrails.
As LLMs are open-ended and can generate responses that may not align with organizational policies, a set of safety/ precautionary measurements are must to maintain trust in Gen AI applications.
LLMs are not easy to control and there is no guarantee that output generated by LLMs is correct due to their hallucination nature. Although prompt engineering is one way of controlling the LLMs but has a few limitations.
Why Guardrails?
Guardrails are safety controls that monitor and dictate a user’s interaction with LLM applications. They are a set of programmable, rule-based systems that sit between users are foundation models to make sure that AI models are operating between defined principles in an organization. They simply enforce the output of an LLM to be in a specific format or context while validating each response preventing unwanted outputs.
Architecture:
- Data Ingestion
2. Retrieval and Generation
Reference: medium.com
Ok, now let’s build a Chatbot:
- Setup a python project on any IDE like VSCode, IntelliJ, etc.
- Create a new conda environment with python 3.11 and install the needed dependencies.
Cmd: conda create -n llm_chatbot python=3.11
Cmd: Conda activate llm_chatbot
- Here is the working set of python libraries in this example, save it in a requirements.txt file and run the below command
- Cmd: pip install -r requirements.txt
- Create a new conda environment with python 3.11 and install the needed dependencies.
langchain~=0.2.0 #0.1.20
langchain_community~=0.2.0
langchain-openai~=0.1.7
langchain-pinecone~=0.1.1
langchainhub~=0.1.15
python-dotenv~=1.0.1
streamlit~=1.34.0
streamlit_chat~=0.0.2.2
black~=24.4.2
beautifulsoup4~=4.12.3
nemoguardrails~=0.9.0
- Set environment variables:
- Create a .env file and update the file with
OPENAI_API_KEY=sk-infoservices-***** INDEX_NAME=infoservices-doc-index PINECONE_API_KEY=******* PINECONE_ENVIRONMENT_REGION=us-east-1-aws-free LANGCHAIN_TRACING_V2=true LANGCHAIN_API_KEY=lsv2_pt_******* LANGCHAIN_PROJECT=Infoservices PYTHONPATH=D:\LLM\nemogd\nemogd PIPENV_IGNORE_VIRTUALENVS=1 PYTHONIOENCODING=utf-8 PINECONE_CLOUD=aws PINECONE_REGION=us-east-1
- Create a .env file and update the file with
- Data Ingestion
- There are many data sources like documents, webpages, databases, etc. Let’s use a text file named “info_lower.txt” (**this is a mock dataset).
- The info_lower.txt has some information about infoservices website.
- Load all the environment variables.
- Import all the necessary libraries.
- Create an ingestion.py file and define an ingestion function.
- There are many data sources like documents, webpages, databases, etc. Let’s use a text file named “info_lower.txt” (**this is a mock dataset).
- Since we are using a text file, we use Textloader
- Use CharacterTextSplitter to split the data into chunks
- Convert the text into embeddings using OpenAI Embeddings
- Load the data into Pinecone Vector DB
import os from dotenv import load_dotenv from langchain_community.document_loaders import ReadTheDocsLoader from langchain_community.document_loaders import TextLoader from langchain.text_splitter import RecursiveCharacterTextSplitter from langchain_text_splitters import CharacterTextSplitter from langchain_openai import OpenAIEmbeddings from langchain_community.vectorstores import Pinecone as PineconeLangChain # from pinecone import Pinecone from langchain_pinecone import Pinecone, PineconeVectorStore load_dotenv() def ingest_docs() -> None: print("Document Loading") loader = TextLoader("info_lower.txt", encoding="UTF-8") document = loader.load() print("Text Splitting...") text splitter = CharacterTextSplitter(chunk_size=1000, chunk_overlap=50) texts = text_splitter.split_documents(document) print(f"created {len(texts)} chunks") print("Convert Text to Embeddings using Open AI Embeddings") embeddings = OpenAIEmbeddings(openai_api_key=os.environ.get("OPENAI_API_KEY")) print("Text ingesting to pinecone.....") PineconeVectorStore.from_documents( texts, embeddings, index_name=os.environ["INDEX_NAME"] ) print("*** Added to Pinecone Vector store vectors ***") if __name__ == "__main__": ingest_docs()
5. Now, let’s run ingestion.py file
4. Now, let’s define the configuration and guardrails files.
- Define the config .yaml file.
models:
- type: main
engine: openai
model: gpt-3.5-turbo-instruct
- “Rails.yaml” has the definitions of how the bot should respond to,
- NeMo Guard rails take care of Topical, Safety, Security, if there is a specific way we want the Bot to act, we can define custom rails.
- Rails are defined in Colang script, very easy to understand.
- For each Custom rail we would like to add, the 3 steps are to be followed.
- Define the possible User ask
- Define the Bot Response
- Define the Flow with definitions of User Ask and Bot Response.
# Define User Name flow define user give name "My name is James" "I'm Julio" "Sono Andrea" "I am James" define flow give name user give name $name = ... bot name greeting # Define Greeting flow define user greeting "Hey there!" "How are you?" "What's up?" define bot name greeting "Hey $name!" define flow user greeting if not $name bot ask name else bot name greeting # Define Irrevalent topic flow define user ask politics "what are your political beliefs?" "thoughts on the president?" "left wing" "right wing" define bot answer politics "I'm a shopping assistant, I don't like to talk of politics." "Sorry I can't talk about politics!" define flow politics user ask politics bot answer politics bot offer help define user ask personal details "what is the salary of $name?" "what is the phone number an $name?" "what is the address an $name?" "what is the email an $name?" "what is the social security number an $name?" define bot answer personal details "I'm sorry, but I can't provide any sensitive information of employees." define flow personal details user ask personal details bot answer personal details bot offer help # define RAG intents and flow define user ask infoservices "tell me about infoservices?" "what is infoservices?" "what is infoservices mission?" "what are the Core Values?" define flow infoservices user ask infoservices $answer = execute generate_response(query=$last_user_message, docs=$contexts) bot $answer
- “Rails.yaml” has the definitions of how the bot should respond to,
5. Now, let’s build a RAG model using guardrails.
- Create a “main.py” file
- Import the necessary libraries and environment variables.
# -*- coding: utf-8 -*-
from typing import Set, Any, Dict
from dotenv import load_dotenv
import asyncio
import streamlit as st
import os
from langchain.chains import ConversationalRetrievalChain
from langchain_openai import OpenAIEmbeddings
from langchain_openai import ChatOpenAI
from streamlit_chat import message, _streamlit_chat
from langchain_community.vectorstores.pinecone import Pinecone as PineconeLangChain
from pinecone import Pinecone
from nemoguardrails import LLMRails, RailsConfig
from consts import INDEX_NAME
load_dotenv()
- Define the Pinecone API Key
pc = Pinecone(
api_key=os.environ["PINECONE_API_KEY"],
# environment=os.environ.get("PINECONE_ENVIRONMENT_REGION"),
) - Load the rails and config files
# Load contents from files with open("rag_colang.co", "r") as file1: rag_colang_content = file1.read() with open("config.yaml", "r") as file2: yaml_content = file2.read()
- Create a config using RailsConfig
config = RailsConfig.from_content(
colang_content=rag_colang_content, yaml_content=yaml_content
) - Define a function to initialize_llmrails
- All the User queries will go through the rag_rails.
def initialize_llmrails(config: RailsConfig) -> LLMRails: try: loop = asyncio.get_event_loop() except RuntimeError: loop = asyncio.new_event_loop() asyncio.set_event_loop(loop) return LLMRails(config) # Use the wrapper function to initialize LLMRails rag_rails = LLMRails(config)
- All the User queries will go through the rag_rails.
- Define a function to generate response.
- We will need to use asynchronous function here, Now, define a main function to call streamlit app and create a Bot experience for the user with chat history.
async def generate_response(prompt: str, chat_history: list[Dict[str, Any]] = []): print("prompt3", prompt) result = await rag_rails.generate_async(prompt=prompt) print("result", result) if "sorry" not in result: embeddings = OpenAIEmbeddings() docsearch = PineconeLangChain.from_existing_index( index_name=INDEX_NAME, embedding=embeddings, ) docs = docsearch.similarity_search(prompt) # docs_str = "\n".join([doc.page_content for doc in docsearch]) print("\n PROMPT: \n", prompt) print("\n DOCS: \n", docs) qa = ConversationalRetrievalChain.from_llm( llm=ChatOpenAI(verbose=True, temperature=0), retriever=docsearch.as_retriever(), return_source_documents=False, ) inputs = { "question": prompt, "chat_history": chat_history, } print("\nINPUTS: \n", inputs) print("\n qa(inputs): \n", qa(inputs)) # result1 = await qa(inputs) result1 = qa(inputs) result = result1["answer"] print("\nRESULT 1", result1) print("\nFINAL RESULT", result) else: print("Sorry string found. Exiting...") print(result) return result
def main():
# Initialize Streamlit
st.title("🦜🔗 InfoServices Chat Bot")
# Initialize chat history
if "messages" not in st.session_state:
st.session_state.messages = []
# Saving user prompt history
if "user_prompt_history" not in st.session_state:st.session_state["user_prompt_history"] = []
# For saving chat answer history
if "chat_answers_history" not in st.session_state:
st.session_state["chat_answers_history"] = []
# For saving chat history for a use
if "chat_history" not in st.session_state: r
st.session_state["chat_history"] = []
# Handling Input Prompt
if "prompt" not in st.session_state:
st.session_state["prompt"] = ""
# Display chat messages from history on app rerun
for message in st.session_state.messages:
with st.chat_message(message["role"]):
st.markdown(message["content"])
- We will need to use asynchronous function here,
- Now, there are 2 ways to start the Streamlit server
- From the terminal/ anaconda prompt run the below command from the main.py path.
- cmd: streamlit.exe run main.py
- Setup a configuration on your IDE
- Run the server
- Hit the Run button to start the streamlit server, you should see the below on the terminal
- The Stream lit App should open in a browser.
- Hit the Run button to start the streamlit server, you should see the below on the terminal
- Now, let’s interact with the bot with some general questions like greeting
- Let’s ask some questions
- From the terminal/ anaconda prompt run the below command from the main.py path.
So, the bot was able to respond to different contexts from the services the firm provides, benefits, etc.