Google’s Ironwood TPUs Explained: The Future of Scalable AI Infrastructure
Drive smarter decisions with next-gen AI acceleration.
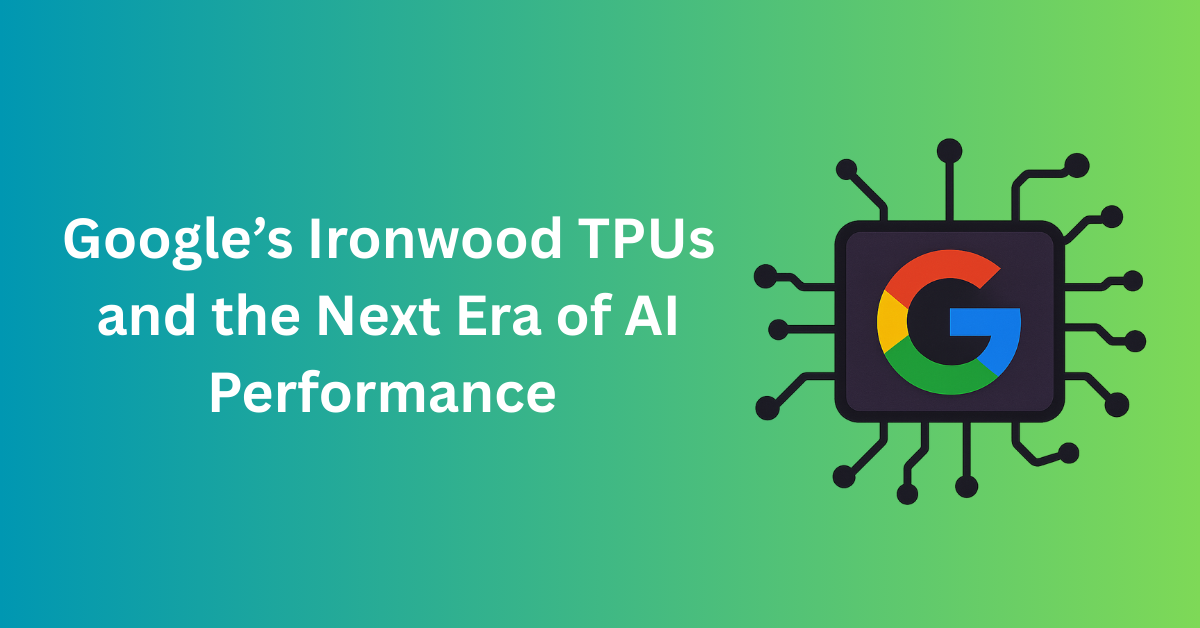
As the AI revolution accelerates, enterprises worldwide seek more powerful, efficient, and scalable ways to run their workloads. In 2025, Google introduced a significant leap forward in AI hardware innovation: the Ironwood TPUs (Tensor Processing Units). These next-generation AI accelerators are designed to meet the escalating demands of modern machine learning (ML) and deep learning (DL) workloads, especially for generative AI, large language models (LLMs), and edge-to-cloud AI ecosystems.
In this blog, we explore Ironwood TPUs, why they matter, how they outperform previous generations, and what they mean for businesses leveraging AI technologies in 2025 and beyond.
What Are Ironwood TPUs?
Google’s Ironwood TPUs are purpose-built processors specifically engineered to accelerate AI training and inference operations with maximum efficiency. Unveiled at Google Cloud Next 2025, these TPUs represent a shift toward exascale computing, enabling businesses to run sophisticated AI models at unprecedented speeds and efficiency.
Ironwood TPUs are the evolution of the TPU v4 and v5e chips and are integrated seamlessly with Google Cloud’s AI ecosystem, including Vertex AI, TensorFlow, and JAX. With improved architectural design, they offer:
- Higher computational throughput
- Reduced training and inference latency
- Greater energy efficiency
- Enhanced memory bandwidth and modular scaling
These capabilities make them ideal for natural language processing (NLP) applications, computer vision, recommendation systems, predictive analytics, and real-time AI-powered services.
Key Innovations Behind Ironwood TPUs
1. Exascale AI Performance
Ironwood TPUs advance AI computing toward exascale performance levels. They can process quintillions of operations per second, making it feasible to train and infer large models such as GPT-4, Gemini, and multimodal AI architectures without bottlenecks.
2. Modular and Scalable Infrastructure
Ironwood chips use a new interconnect architecture that supports modular scaling across cloud and hybrid environments. This allows enterprises to scale AI workloads horizontally without redesigning infrastructure from scratch.
3. AI Sustainability by Design
Aligned with Google’s commitment to carbon neutrality, Ironwood TPUs are engineered for energy-efficient AI computation. They use more innovative scheduling, optimized silicon layout, and thermal-aware design to reduce power consumption per workload.
4. End-to-End Ecosystem Integration
Ironwood TPUs integrate natively with Google Cloud AI tools such as:
- Vertex AI Pipelines for orchestration
- TensorFlow and PyTorch for development
- MLOps tools for lifecycle management
- AI security and data governance features
Understanding the Architecture: How Ironwood TPUs Work
Ironwood TPUs' core is a custom-designed architecture optimized for matrix-heavy operations commonly used in deep learning models. Here's a breakdown of its working components and flow:
1. Matrix Multiply Units (MXUs)
Ironwood TPUs feature advanced MXUs, which are specialized for performing massive matrix multiplications—the backbone of neural network computations. These MXUs allow multiple layers of a model to be computed in parallel with minimal memory bottlenecks.
2. Unified High-Bandwidth Memory
The chips include unified, high-speed memory with increased bandwidth compared to TPU v4. This reduces the latency in accessing training data and model parameters, which is crucial for large model execution.
3. Custom Interconnect Fabric
One of the defining traits of Ironwood TPUs is the custom-designed optical interconnect fabric that links hundreds (or thousands) of TPU chips together. This allows for:
- Faster communication across nodes
- Support for model parallelism (splitting one model across many TPUs)
- Efficient data parallelism (running multiple copies of a model on different inputs)
4. TensorFlow XLA Compiler Integration
Ironwood TPUs work closely with the XLA (Accelerated Linear Algebra) compiler, which optimizes standard ML code for TPU hardware. This abstraction allows developers to write in familiar frameworks (like TensorFlow or JAX) while letting the compiler optimize execution for Ironwood.
5. Smart Scheduling and Thermal Management
Ironwood leverages AI-driven workload scheduling and thermally optimized chip placement to enhance system performance while reducing heat output and energy usage. This makes it ideal for high-density data centers and green computing environments.
Why Ironwood TPUs Matter for Enterprise AI
1. Faster AI Model Development
Traditional model training cycles can take days or even weeks. With Ironwood TPUs, businesses can drastically reduce training time, from days to hours, enabling quicker iterations, testing, and deployment.
2. Reduced Infrastructure Costs
Efficient computing means reduced resource consumption. Companies can achieve better performance per dollar, making advanced AI affordable even for mid-sized businesses.
3. Time-to-Market Advantage
By minimizing model development and deployment timelines, Ironwood TPUs help businesses release AI-powered products and features faster — a key differentiator in today’s digital-first economy.
4. Supports Complex AI Architectures
From transformer-based LLMs to multimodal networks and federated learning systems, Ironwood TPUs can handle diverse, compute-heavy AI architectures with ease.
Ironwood TPUs and the Rise of Agentic AI
With Ironwood TPUs powering more complex AI systems, we’re entering what Google calls the “agentic AI era.” Agentic AI refers to intelligent systems that can perform autonomous tasks, adapt based on feedback, and collaborate with other systems or humans.
A key enabler of this shift is Google’s Agent Development Kit (ADK)—a toolkit designed to help developers build, deploy, and manage AI agents that can take meaningful actions.
Ironwood TPUs provide the processing backbone to run these intelligent agents at scale, while ADK delivers the software framework to orchestrate them. From smart chatbots to autonomous research tools, this duo could reshape how enterprises apply AI.
Industry Use Cases: Ironwood TPU in Action
Industry | AI Use Cases |
---|---|
Healthcare | - Faster genomic sequencing using deep neural networks - Real-time diagnostic imaging for personalized medicine - Predictive analytics in public health and drug discovery |
Finance | - AI-powered fraud detection with lower latency - Automated risk assessment and portfolio optimization - NLP for financial document analysis |
Retail & E-Commerce | - Smarter recommendation engines - Real-time customer behavior analytics - Demand forecasting and inventory management |
Manufacturing | - AI-driven quality control systems - Predictive maintenance using sensor data - Edge-to-cloud AI for robotics and automation |
Media & Entertainment | - Faster video and image generation - Automated content moderation - Personalized user experiences via AI recommendation systems |
Strategic Advantage for 2025 and Beyond
Future-Proofing Your AI Strategy
Ironwood TPUs aren’t just built for today’s AI demands—they’re designed to power the breakthroughs of tomorrow. As new models become more complex, having an AI-first infrastructure becomes a key competitive advantage.
Leveraging GCP's AI Stack
Businesses integrating Ironwood TPUs with GCP’s AI stack (Vertex AI, BigQuery ML, AutoML, etc.) gain end-to-end visibility, control, and performance across the full AI lifecycle.
Conclusion: The Future is Powered by Ironwood
As AI matures into the backbone of digital transformation, the hardware supporting it must evolve too. Google’s Ironwood TPUs mark a significant milestone in that journey, offering not just performance but a full-stack solution for scalable, sustainable AI.
FAQ'S
1. What are Google Ironwood TPUs?
Google Ironwood TPUs are next-gen AI accelerators developed by Google to boost machine learning and deep learning performance in scalable cloud environments.
2. How do Ironwood TPUs improve AI performance?
They offer faster compute power, reduced training time, and better efficiency for large-scale AI models like LLMs, making them ideal for high-performance AI tasks.
3. What makes Ironwood TPUs different from earlier TPU versions?
Ironwood TPUs provide higher throughput, better energy efficiency, and more seamless integration with Google Cloud compared to earlier versions like TPU v4.
4. Who can use Ironwood TPUs on Google Cloud?
Startups, enterprises, and research institutions can access Ironwood TPUs via Google Cloud to scale their AI workloads affordably and efficiently.
5. Are Ironwood TPUs only used for deep learning?
No. While ideal for deep learning, Ironwood TPUs also support other AI workloads like NLP, computer vision, and generative AI across various industries.
6. How do Ironwood TPUs enable scalable AI infrastructure?
They allow distributed computing at scale, letting organizations train massive models faster while maintaining energy efficiency and reducing operational costs.